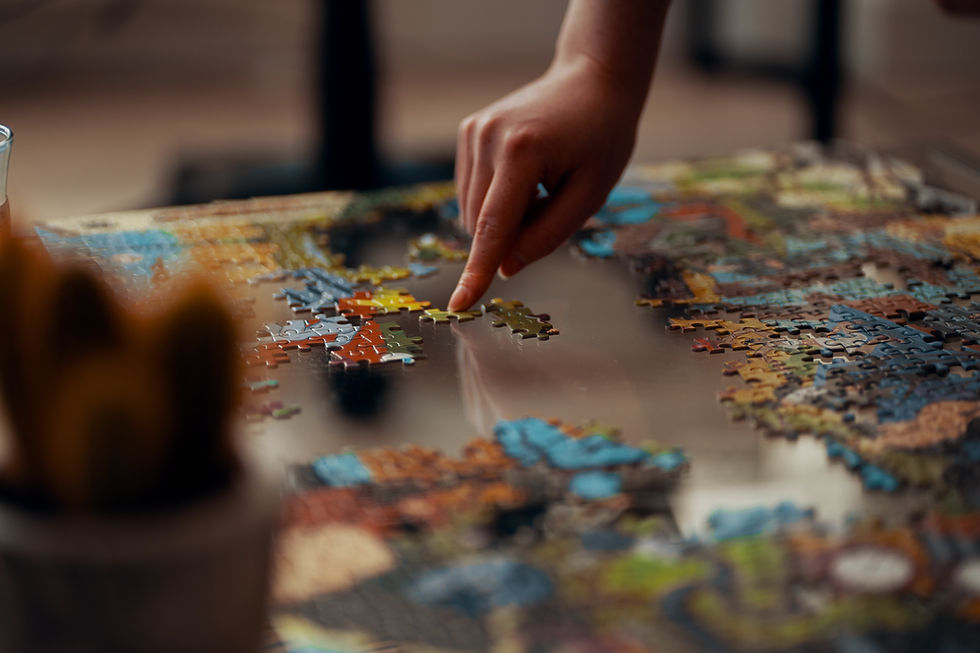
Education is often focused on the direct transmission of information from teachers to students—a chalk-talk lecture explaining key historical events or a textbook presenting mathematical formulae. However, research shows active construction of knowledge through inferential thinking results in deeper, longer-lasting learning. As emerging AI capabilities advance, technology holds promise for enhancing inferential learning by providing optimal scaffolds for productive reasoning rather than overt answers. By combining AI’s endless patience and adaptability with the meaningfulness of reaching conclusions through mental effort, we can unlock learning that is both efficient and profound.
The Science Behind Inferential Learning
Inferential learning centers on learners inductively making meaning for themselves by observing patterns, testing hypotheses, and drawing conclusions based on available evidence. Rather than passively receiving facts and procedures, students actively integrate new experiences with prior knowledge to build mental models and form logical rules.
Cognitive and learning sciences reveal this knowledge construction through inductive inference and exploration fundamentally aligns with how our brains naturally gain understanding. The mental effort involved in analyzing information and making connections cements the learning at deeper levels of comprehension and retention. Correctly inferring concepts provides rewarding feedback that motivates ongoing inquiry.
Additionally, exercising inferential thinking itself strengthens core cognitive abilities such as pattern recognition, critical analysis, logic, and creative problem solving—crucial skills both in academics and real-world settings. Inferential learning develops the capacity to make sound judgments in future situations where explicit guidance is unavailable.
Problems with Direct Instruction Approaches
In contrast, direct instructional models often focus solely on explicit top-down transmission of isolated facts, procedures, or theorems with limited broader context. This overly explicit information delivery encourages surface-level rote memorization rather than meaning-making. Students cram for exams and then purge as much content from memory as soon as possible afterwards.
Even clear explanations by expert teachers often fail to inspire deep processing and integration. When students are “told the answer” rather than developing insights themselves, it deprives them of the productive mental struggle that cements learning. Overtly providing solutions is tempting for teachers aiming to accelerate progress, but eliminates the reasoning effort essential for comprehension.
And passive, lecture-based direct instruction tends to lose student interest quickly, especially in our era of short attention spans and information overload. Current estimates suggest 10-20 minutes is the maximum duration students can maintain focus during teacher-centered verbal explanation. Traditional direct instruction scales poorly.
The Power of Productive Struggle
Decades of findings from learning science research, however, reveal that allowing students to productively grapple with new concepts and make inferences themselves before receiving explicit teaching optimizes both engagement and retention. People learn best when solving challenges just beyond their current competence with appropriate scaffolding and feedback.
Having to actively connect ideas and evaluate evidence to reach conclusions exercises and strengthens the very cognitive skills educators aim to cultivate—skills that persist beyond schooling. Guided inferential learning also builds student persistence, curiosity, and capacity for overcoming obstacles through effort. Students gain confidence in confronting ambiguity and reasoning through uncertainty.
Skilled teachers have long understood the art of providing minimal guided assistance at optimal times to spur generative learning, while resisting the urge to explain everything immediately. With the right balance of scaffolds, students construct knowledge most meaningfully through their own mental effort. Now with AI, we can provide that “optimum minimal” guidance at full scale.
How AI Can Unlock Inferential Learning
Recent exponential progress in artificial intelligence offers exciting potential to unleash inferential learning across educational contexts by providing adaptive scaffolding tailored to each individual learner’s needs—exactly when they need just enough help to stay productively challenged. A few examples of how emerging AI capabilities can promote inference:
- Adaptive learning systems generate personalized sequences of inquiry-driven activities with carefully timed hints and prompts based on demonstrated learner needs.
- Intelligent conversational agents ask probing Socratic questions and have learners justify conclusions without confirming the answers explicitly.
- Virtual simulations allow students to gather evidence inductively and derive patterns and theories themselves with adaptive guidance.
- Powerful machine learning algorithms can create endless novel inferential challenges at appropriate difficulty levels for full mastery.
- Chatbots engage students in natural language dialogues that require demonstrating their reasoning while avoiding overt exposition.
In essence, AI expands teachers’ ability to craft the optimal path for students to uncover concepts and construct mental models through effortful inferences. Automating time-consuming manual scaffolding enables this kind of deep inferential learning at full scale.
Time-Saving Benefits
In fact, one major advantage of AI-powered inferential learning is the potential time savings versus direct instruction. Eliminating lectures and lengthy explanations, and instead providing rapid, adaptive scaffolding as learners actively figure concepts out themselves results in large time savings:
- Students proceed faster by focusing mental energy on productive reasoning rather than passive listening.
- Flipping lectures into pre-class inference activities frees more in-class time for higher-order work.
- Misconceptions get addressed flexibly in real-time rather than re-teaching entire lessons.
- Students needing more inference practice get it instantly without holding the class back.
- Teachers avoid repetitively delivering the same explanations every class period, freeing up their time.
Automating scaffolding enables students to truly master concepts through inference in significantly less time than via traditional methods. Efficiency plus depth.
Subject-Specific Examples
Educational contexts ripe for enhanced inferential learning via AI span diverse subjects and age groups:
Mathematics: Rather than providing derivations, have students analyze patterns in datasets, derive general formulae themselves, and prove their conjectures. The AI supplies just-in-time scaffolding personalized to each learner.
Science: Students actively investigate simulated worlds, gather observations, and inductively develop explanatory models and theories. Adaptive AI hints prevent unproductive theorizing.
History: By wrestling with analysis of primary sources, archeological evidence, and other relics of the past, students construct rich inferences about historical eras, events, and figures. AI nudges them to draw sound conclusions.
Literature: Instead of pre-interpreting symbolism and themes for students, AI systems craft Socratic questioning and analysis activities that let learners develop original evidenced interpretations themselves.
Medical Education: Future clinicians are immersed in VR patient simulations and must infer diagnoses and treatment plans through active investigation, with AI guidance on developing clinical reasoning expertise.
The Teacher's Vital Role
Importantly, AI is not about replacing teachers, but rather empowering them to facilitate more active, transferable learning. Educators maintain essential roles:
- Establishing learning goals, sequences, and resources. AI does not set objectives.
- Designing conceptual discovery activities and acquiring rich immersive resources.
- Training students in effective, ethical inferential and inquiry strategies.
- Continually evaluating student progress using AI-assisted assessment.
- Providing human social-emotional support, motivation, and celebration of successes.
Additionally, teachers can focus more class time on higher-order cognitive activities like collaborative projects, design challenges, and reflective discussion enhanced by students’ foundational knowledge acquired via inference. AI amplifies human educators’ abilities.
Addressing Potential Objections
Integrating inferential learning powered by AI will naturally face obstacles and objections:
- Misconceptions about entirely unguided discovery learning: This approach stresses strategic scaffolding not pure exploration. Teachers set goals.
- Fears about AI replacing human teachers: AI will enhance not replace educators in guiding inferential learning. Unique human strengths remain crucial.
- Concerns about current technological capability: While still evolving, rapid advances make AI scaffolds increasingly able to optimize inference. Used judiciously now, adoption will expand.
With continued development and thoughtful implementation, AI-supported inferential learning can mitigate these concerns and realize immense educational benefits.
Implementation Recommendations
To integrate AI aids for inference successfully:
- Phase in gradually, while providing teachers with ample training on effective strategies for guiding inferential learning pathways.
- Start with supplemental low-stakes practice to build user familiarity before higher-stakes applications.
- Continuously gather student outcome data, solicit user feedback, and upgrade system scaffolds and interface usability iteratively.
- Focus first on skills most naturally learned through guided inference like mathematical reasoning and scientific thinking.
With an evidence-based foundation and iterative mindset, schools can thoughtfully harness AI to enhance meaningful inferential learning.
The Exciting Potential Ahead
The possibilities of combining active student inquiry with tireless, adaptive AI support are truly exciting. We envision:
- Students intrinsically motivated by the rewards of figuring out concepts themselves.
- Learners developing lasting cognitive capabilities to tackle novel challenges.
- Flexible systems customized to students’ diverse needs and paces.
- Teachers freed to focus on human mentoring and inspiration.
- Lifelong skills in analytical, critical thought and sound judgment.
By supporting learners to actively construct understanding guided by optimal, personalized AI scaffolding, education stands to make great leaps forward in engagement, outcomes, and preparation for lifelong learning and work. The future is active.
More Effective Learning for All
Inferential thinking develops deep, durable learning critical in our complex world. AI’s endless capacity for timely, customized scaffolding paired with education’s focus on meaningful knowledge construction signals a powerful combination. Although still early, rapid advances bring this future steadily closer. When used judiciously under teachers’ direction, AI-enhanced inference promises more engaging, efficient, and empowering learning for all.
Comments